A Comprehensive Guide to Implementing Data Governance for AI
Data governance and AI are deeply intertwined.
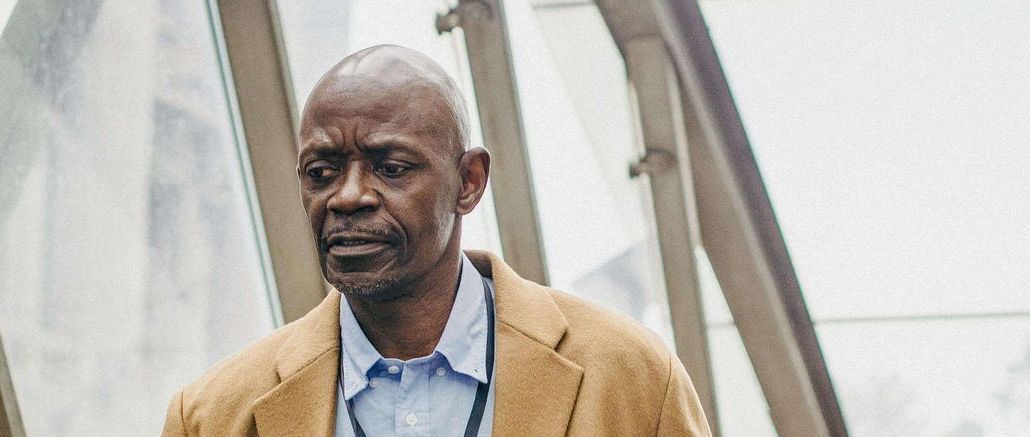
As artificial intelligence (AI) becomes increasingly integral to business operations, a few of you have been talking to me about the importance of robust data governance. As you know, AI platforms rely on vast amounts of data to function effectively, and without proper governance, organisations risk compromising data quality, security, and ethical standards. This guide will help you navigate the complexities of data governance for AI, offering practical steps for implementation and aligning with UK regulatory frameworks.
Data governance and AI are deeply intertwined. AI systems depend on large datasets to learn and make decisions. The quality, integrity, and ethical use of this data directly impact the outcomes of AI models. Therefore, implementing strong data governance is crucial for ensuring that AI systems are reliable, fair, and compliant with legal and ethical standards.
There are 2 key aspects where data governance and AI intersect: data quality and compliance. It’s a given that high-quality data is essential for training accurate and reliable AI models but regulatory requirements, such as the UK’s GDPR, demand strict controls over how data is collected, processed, and used, especially in AI systems. Data governance frameworks must therefore incorporate ethical considerations, such as:
transparency (stakeholders should be informed about the nature of the data, the purpose of its use, and the logic behind AI decision-making processes)
fairness (think about regularly auditing datasets for representativeness and bias, as well as implementing fairness metrics in the AI model evaluation process)
accountability (this includes assigning responsibility for data governance, monitoring AI system performance, and ensuring there are mechanisms in place to address and rectify any issues that arise, such as erroneous or harmful AI decisions)
data minimisation (collecting only the data necessary for specific AI functions which in turn reduces the risk of misuse and enhances privacy protections).
To recap, you need to ensure, the data is fit for purpose, ethical standards are upheld and regulatory compliance is maintained as well as ensuring that data security is enforced. This step-by-step checklist should help get you on the right track.
Step 1. The first thing to do is to assemble a cross-functional data governance team
Why? A successful data governance initiative requires expertise from various domains, including data science, IT, legal, compliance, and business operations.
How? Form a governance committee that includes representatives from each of these areas. This team will be responsible for developing policies, overseeing implementation, and ensuring ongoing compliance with data governance and AI ethics standards.
Step 2. Define Data Governance Objectives and AI-Specific Principles
Why? Clear objectives and guiding principles help make sure that data governance efforts are aligned with organisational goals and AI-specific needs.
How? Discuss and define objectives that focus on maintaining the 3 key pillars of data quality, protection of sensitive data, and ensuring ethical AI use.
Some suggested areas to consider include:
Fairness: AI models should be free from bias and should not discriminate against any group.
Transparency: AI decisions must be explainable and understandable to all stakeholders.
Accountability: There should be clear accountability for AI-driven outcomes.
Step 3. Conduct a Data Audit with an AI Focus
Why? Understanding your data landscape is crucial for identifying gaps that could affect AI model performance or ethical use.
How? Audit your data sources, focusing on the quality, accuracy, and representativeness of the data used in AI training. Identify any potential biases and assess the security of sensitive data.
Step 4. Develop Data Governance Policies Tailored for AI
Why? AI platforms have unique requirements, and governance policies must reflect these needs.
How? Draft policies that cover:
Data collection and usage ensuring data used in AI models is collected with proper consent and is relevant to the AI’s purpose.
Bias detection and mitigation implementing processes to regularly check AI models for bias and take corrective actions.
Model transparency establishing guidelines for documenting AI models and ensuring their decisions are explainable.
Data access controls, defining who can access data, especially sensitive information used in AI, and under what conditions.
Step 5. Implement AI-Specific Data Governance Tools and Processes
Why? Technology is essential for enforcing data governance policies effectively.
How? Invest in tools that support data cataloguing, data quality management, and bias detection in AI models, such as data catalogues, data profiling tools, and AI fairness toolkits.
Examples of these might be:
Data Catalogues: Tools like Collibra or Alation help organisations create and maintain an inventory of data assets, making it easier to track data lineage, manage metadata, and ensure consistent data usage across AI projects.
Data Profiling and Quality Management: Solutions like Talend or Informatica allow for the assessment and improvement of data quality. These tools can automatically detect anomalies, cleanse data, and enforce quality rules, ensuring that AI models are trained on accurate and reliable data.
Bias Detection and Fairness Toolkits: Tools such as IBM’s AI Fairness 360 or Google’s What-If Tool are specifically designed to identify and mitigate biases in AI models. These tools provide metrics and visualisations that help in assessing fairness, allowing organisations to take corrective actions when necessary. Then make sure you are doing regular data quality checks, bias audits, and AI model transparency assessments. Ensure these tools and processes are integrated into your AI development lifecycle.
Importantly, the following is then advised.
Step 6. Establish Training and Awareness Programs
Why? Employees need to understand the intersection of data governance and AI ethics.
How? Develop training programmes that cover data governance policies, AI ethics, and legal requirements (such as UK GDPR). Other ideas include running regular workshops, e-learning modules, and putting out internal communications to ensure that all employees are aware of their responsibilities.
Finally,
Step 7. Monitor, Review, and Update Your Data Governance Framework
Why? AI and data governance are dynamic fields, requiring continuous improvement.
How? Set up a system for ongoing monitoring of AI models to ensure compliance with data governance policies. Conduct regular reviews and update your framework as new risks, technologies, or regulations emerge. Engage with external auditors to validate your practices.
Optional extra
Step 8. Engage with External Stakeholders and Regulatory Bodies
Why? Transparency and accountability are essential for building trust with stakeholders.
How? Regularly communicate your data governance practices to external stakeholders, including customers, partners, and regulators. Stay aligned with UK regulatory guidance from bodies like the Information Commissioners Office (ICO) and Centre for Data Ethics and Innovation (CDEI). Also you might consider publishing transparency reports that outline your AI practices and data governance efforts.